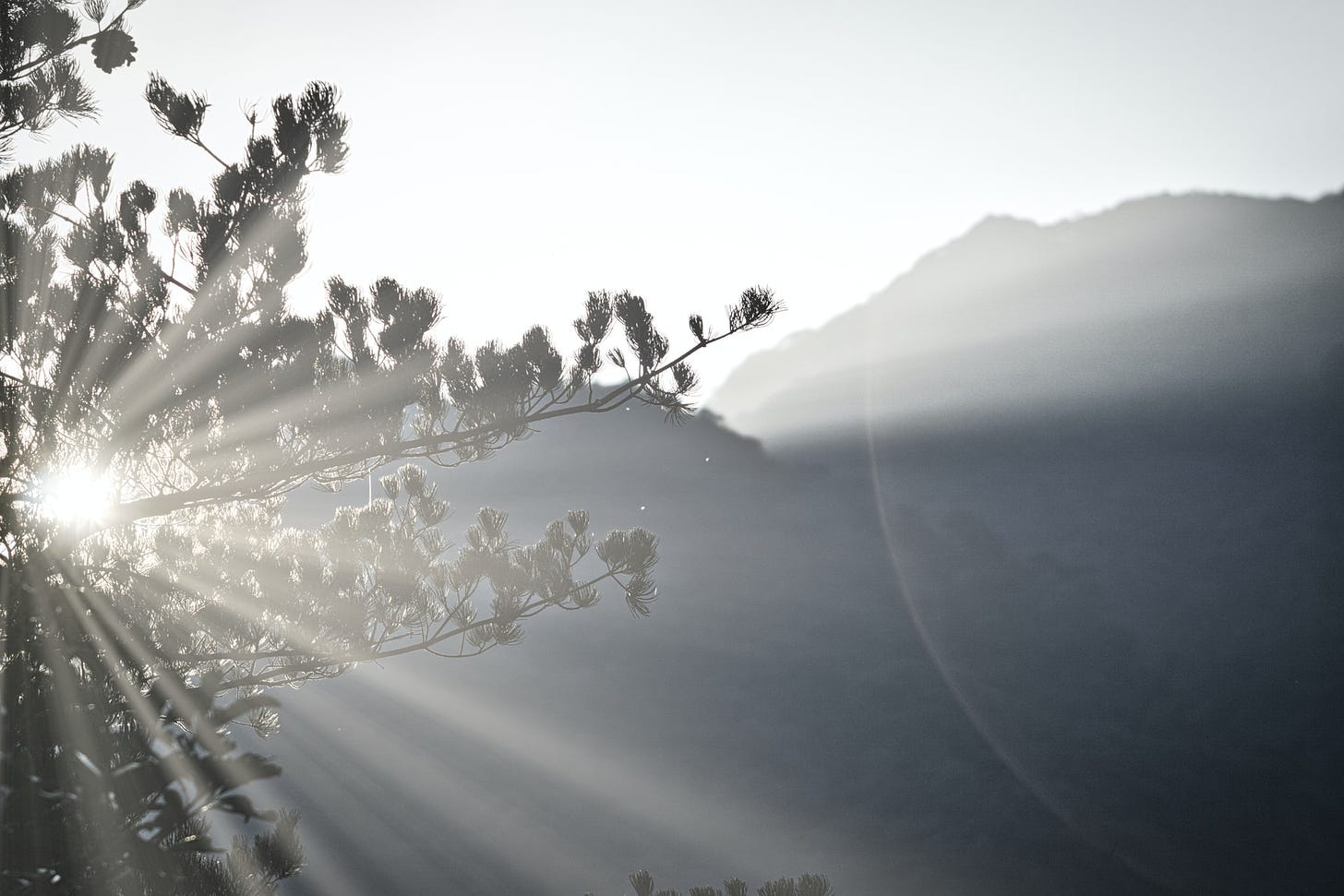
Hey there! I realize that there are a ton of blog posts and resources out there for getting started in data science and machine learning. Personally, I transitioned from academia to data science over three years ago. Since then, I have read various opinions on how best to learn data science, and I have taken or perused a number of online courses.
Based on this experience, I would like to summarize courses and resources I have found the most useful. I will do this over the course of several posts. In this post, I highlight resources if you are starting from scratch with data science. In a follow-up post, I will write about some additional courses that are sort of off-the-beaten-path, but may appeal to you, depending on your unique background.
Also, if you are not a beginner, I still recommend checking out the book recommendation below. It is a great resource for both beginner and seasoned data scientists alike.
Start Here --> Andrew Ng's Classic Machine Learning Course
You may have already heard of this course, and in my view, that can be a good thing. When you start applying for jobs, and say, "I've taken Andrew Ng's machine learning course", they will likely have heard of it and will know what you have learned.
This course starts from the basics of linear regression and works up to neural networks and unsupervised learning algorithms (it will also explain what supervised and unsupervised learning means, if you haven't been exposed to that yet). The course gives a taste of the mathematics behind the algorithms, without being too overwhelming for those without an extensive math background (or, for those for whom it's been a few years since they last took a math course!).
When taking this course, I would recommend working through the homework coding assignments, either as you go or after you've gone through all the lectures. These coding assignments give you a taste for what is going on "behind the scenes" when you are running your own machine learning models later on.
Reading Companion --> The Hundred-Page Machine Learning Book
I find that when learning something new, it helps to read about the same concepts described in different ways, by different people. Andrew Ng does a nice job describing the foundational concepts of machine learning, but the course is a little old, and maybe you are interested in being exposed to some newer ideas.
The Hundred-Page Machine Learning Book is a fairly easy read. It focuses on the concepts, without getting bogged down with the math. It is the only resource I have seen, so far, that summarizes all of the main concepts in machine learning, from linear regression to how to deal with imbalanced datasets to newer ideas like one-shot learning, all in one place. There is also a companion wiki with resources to go to if you want to take a deeper dive into one of the topics touched upon in the book.
Because this book covers such a broad range of topics, it can serve as a good resource for even the seasoned data scientist when working on a new project or when looking for some new ideas when you are stuck.
Bottom Line: If you take Andrew Ng's course, and read this book, you will have a very solid foundation in machine learning and will be ready to move on to some more advanced techniques and ideas.
The Job Search
It is likely that, if you are reading this, you are either applying for jobs or maybe you will find yourself applying for a new job in the future. Occasionally, I will include at the bottom of this newsletter some job search or interview tip.
I will close this week's newsletter with probably the most common interview question there is:
Tell me about yourself.
I guess this is more a statement, then a question, but you get the idea.
Think about your answer to this question, and then check out these resources to (hopefully) help you tackle this question more confidently:
Enjoy the rest of your week!