[#3] Off the Beaten Path
Valuable online courses related to data science that you may not have heard of
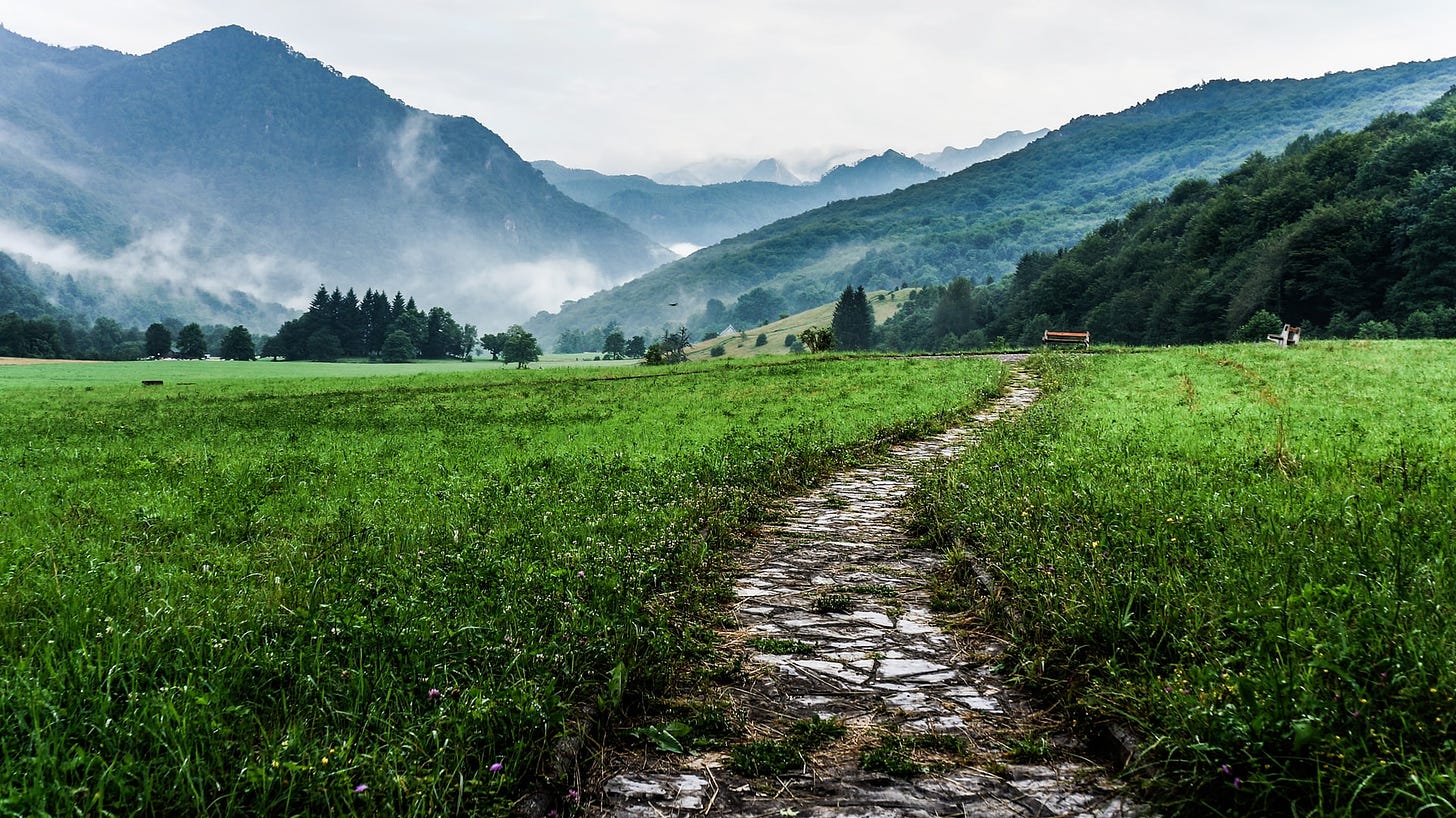
There are many websites and bootcamps and Coursera courses that want to teach you data science. In a previous e-mail, I recommended the classic Andrew Ng Machine Learning Coursera course and The Hundred-Page Machine Learning Book. It's important to start with the fundamentals and then decide where you want to go from there.
I have a few "off-the-beaten-path" courses to recommend. If you don’t have time right now for another course, I also recommend below some specific videos to review some important concepts.
Some of the resources below can be useful, not just for beginners, but also for more experienced data scientists, who might be looking to refresh their knowledge in certain areas or fill in some gaps in their knowledge.
First, I want to highlight several courses that are not necessarily all data science courses, but they cover some important concepts that a data scientist (or a data-scientist-in-training) might want to review.
Statistics
If you are looking for a quick overview of statistics, or just need a refresher, these lecture notes from an NYU statistics course may be helpful. You probably don't need to go over all of the proofs, but understanding some of the key concepts is important for a data scientist.
If you have not had any formal education or training in statistics and really want to dive deeper, then check out a Coursera specialization called Advanced Statistics for Data Science.
Some of the lectures in this specialization may be overkill, but you should be conversant in topics such as P-values, the central limit theorem, and hypothesis testing. Here are some specific lectures to pay attention to, if these topics are a little fuzzy for you:
Mathematics
Behind the scenes of most machine learning and AI algorithms is some complex (and very interesting) math. If you are interested in learning more about this math (or if it's been a while and you're looking for a refresher), then I recommend the Mathematics for Machine Learning Specialization on Coursera.
This specialization consists of three courses. The first course is Linear Algebra (think, vectors and matrices), and then the second course is multivariate calculus. At this point, they discuss how these concepts are used in training neural networks, as well as linear regression models. Finally, the third course in the specialization works up to deriving the PCA (principle component analysis) algorithm.
I found the first two courses to be excellent, with relevant practice problems and providing good intuition behind the different concepts. The third course was a little bit harder to follow and the assignments are not as well thought out (you can see from the reviews on Coursera that this is a common sentiment among people who have taken this specialization). It has been at least a year since I took this course, so they may have made improvements in the meantime.
Machine Learning
If you are interested in a course that approaches machine learning a little differently than in other courses I have seen, in particular, through the lens of statistics, check out Foundations of Machine Learning, taught by David Rosenberg, a data scientist in the Office of the CTO at Bloomberg.
If you don't have time to go through the whole course, you can find all of the lecture slides, and even homeworks and solutions, posted on the course website. You can peruse the slides, and find topics that interest you and focus on just those lectures. Also, there are several videos, in particular, that I highly recommend viewing:
Lecture 7 - Lasso, Ridge, and Elastic Net: I found this video particularly interesting. It illustrates the effect of having correlated features, when using different types of regularization, in particular how these correlated features are weighted in the trained model. This has implications for "explainable AI", which is a topic I plan to focus on in future posts.
Lecture 14 - Performance Evaluation: What I like about this lecture is how he discusses the way to frame your results. If you train a model and get, say, 80% accuracy or true positive rate (or whichever metric is relevant to your case), how do you know if that is a good result, and perhaps more importantly, how much harder should you work to reach some higher accuracy? In this lecture, he talks about "baseline" models and "oracle" models, which can be used to frame the kind of accuracy, or other metric, you can expect given the task and the data in hand. Furthermore, this lecture goes over some of the commonly used metrics, especially for classification problems, and does a good job introducing and explaining them.
Neural Networks
My experience with neural networks is more limited than with other flavors of machine learning, so I do not have a specific recommendation for a course that focuses primarily on neural networks. Several courses that I have looked at, but I have not completed in full, are the following:
Introduction to Machine Learning by Duke University
Deep Learning Specialization by deeplearning.ai
From the first course, I enjoyed this discussion of model selection and the bias-variance trade-off and also this video on the history of neural networks.
Final Thoughts
Whether you are just starting your journey in data science or have been working in data science for a while, I hope that you have found some resources in this post that are useful for you. If you a beginner, I don’t want you to feel that you have to go through all of these courses and be an expert in all of the topics covered here. It depends more on your specific interests and what type of data science role you are in (or hope to be in). So, pick a course that looks interesting to you and enjoy!
The Job Search
It is likely that, if you are reading this, you are either applying for jobs or maybe you will find yourself applying for a new job in the future. Occasionally, I will include at the bottom of this newsletter some job search or interview tip.
In the various job interviews that I have had for data scientist roles, I have been asked quite a range of questions on statistics, probability, machine learning, and even programming / software development.
Here are two statistics questions I have been asked:
What is a P-value?
Define the Central Limit Theorem.
I was asked exactly these questions (in two different interviews). Some interviewers want to know how comfortable you are with statistics, so they will choose one or two statistics concepts to quiz you on. If you watch the videos from towards the beginning of this post, you should be able to give an answer to these two questions.
If you still want further, or an alternate, explanation on these concepts, then check out these resources:
For the P-value, take a look at these lectures on hypothesis testing [this link may require logging in to Coursera, but the videos and lecture notes can be viewed for free].
Enjoy the rest of your week!